How to build data science platforms - Part 5: Data visualization and reliable results
Reading time: approx. 2min
What does a modern data science platform need to offer companies real added value?
Contradiction and connection at the same time: information content vs. simple presentation
A meaningful result or the answer to important questions is not everything. Making data and results comprehensible is just as important for companies as target-oriented analysis1. The more complex a situation is, the more difficult it becomes to present the necessary information in an easy way. But that’s exactly what platforms have to face these days: To present the abundance of data and the results in an understandable way. This is a difficult undertaking. Especially when distributing information to non-experts, it is important to make the gained knowledge comprehensible. The density of information and a presentation that is not overburdened is an art. Everyone who has ever created an infographic knows that. However, this is exactly what an data science platform must deliver.
It is particularly important that no false impression is created even with a simple representation: Disproportionate scale ratios, incomprehensible axis labels, a poorly distinguishable choice of color and many other factors can lead to misunderstandings, an unintentional distortion of results and incorrect interpretations. In this context, other aspects need to be considered – we will discuss this in the next paragraph.
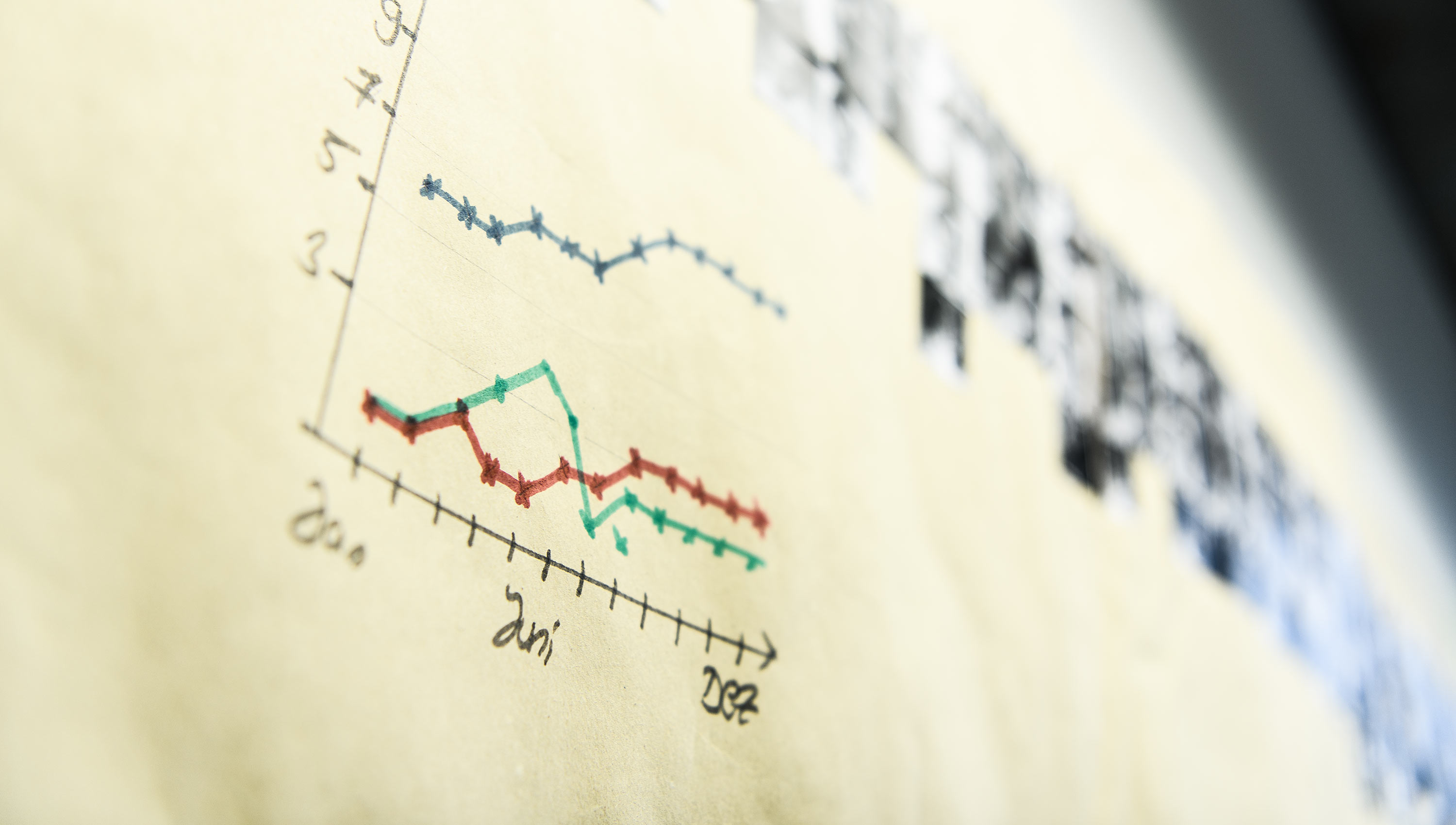
Visualization of resilient results, acceptance and trust
If data is visualized, the next question arises: How can companies rely on the results? Often human competence has to be relied upon. If the results of the analyses are sent back and forth between different applications, Excel sheets or content systems, edited, returned, released and re-sent, there must inevitably be additional control mechanisms to ensure the accuracy of the data. Accordingly, there is an additional effort.
One consideration of how this could be done would be to have the environment in which the various analyses take place provide a visualization of the results at the same time. If the platform traces back each data point to the actual data sources, the people who present the results can not only act faster, they can also provide information to others without additional reinsurance. In this way, the analysis environment would also significantly improve the flow of information within the organization. For example, the results of analysis projects could be displayed in real time on screens at different locations. With reference to the previous section, different screen sizes and ratios also need to be considered. Small screens compress, wide screens lengthen. This can lead to false impressions. This shows which factors must be considered with a genuine scalable solution.
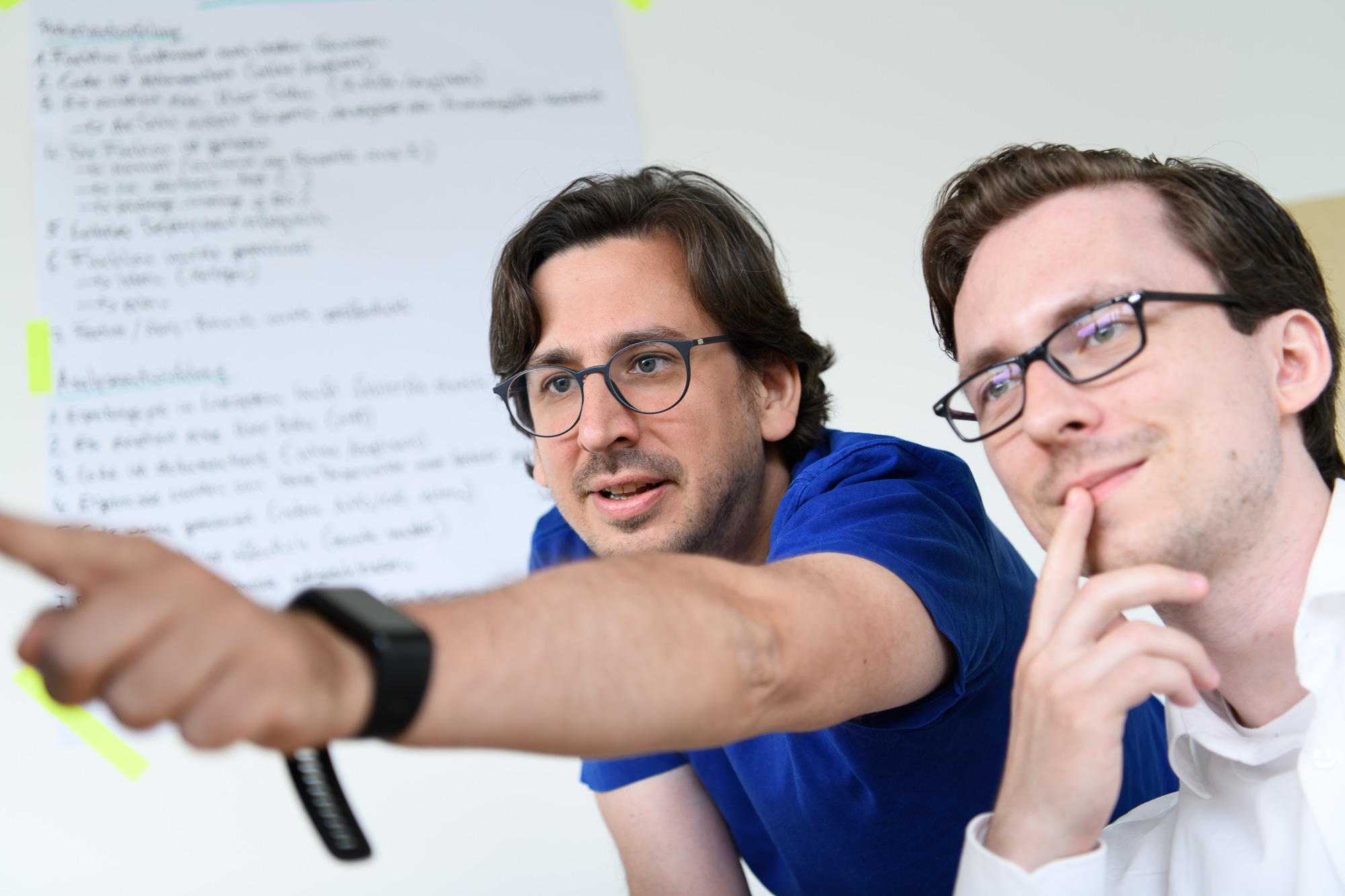
CONCLUSION: If a data science platform should be used company-wide, it must not be measured exclusively by the use of analyses. Especially the “non-scientific” aspects are of high relevance, so that all participants in the company can profit from the generated benefits.
Previous articles
- UI & Teams
- Intelligent user and role concept
- Individualized workflows and dashboards
- Database scalability and business models
1 BARC GUIDE, BUSINESS INTELLIGENCE & BIG DATA 2019 (Printed edition), Page 10